CEO SERIES: Deploy
How Rapid Deployment Can Save Your AI Application from a Slow Catastrophe
Natural Intelligence teaches Artificial Intelligence the #1 method to succeed in the field
I am writing this post while watching my fourth child, newborn Leonardo Versace, take a nap on his fifth day of life.
As with all babies I have heard about or watched in action, Leonardo came to life with a very basic set of skills and a bit ‘undercooked’. He will not know how to walk, talk, let alone make a cappuccino for his dad, for several months (by two years old, he should be a skilled barista).
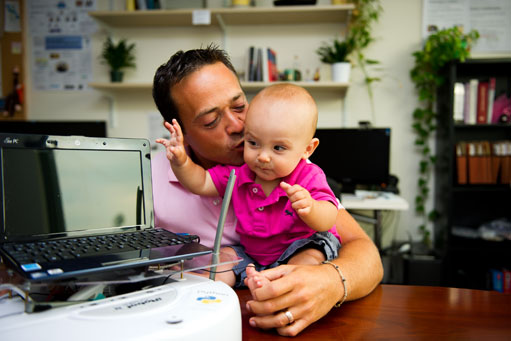
Mother nature chose a very peculiar development method for humans. Our babies come to life with a very limited, though impressive, repertoire of basic skills, with one above all: learning.
Then, iterate and continually learn on the field day-after-day.
Humans are innately built with the ability to build basic skills, learn and develop beyond their initial understanding of the world, and deploy their skills in the real world. Babies eventually get to the point where they can perceive and grasp robots and rip them apart (see image above, with my firstborn Gabriel about to tear off various pieces of the unfortunate iRobot in front of him).
If we could follow our own footsteps in building and deploying AI, we would prevent many of the problems enterprises usually face. The reality is that most AI development follows a very different process than the one outlined above. Product Managers and Engineering teams tend to build as much as they can of the AI system before even attempting to test it in a relevant environment. The basic, ill-fated idea is: “let me put as much AI in the system as I can, I know what it needs to do, and then when I am done 67 months later, fingers crossed it works!”.
Then, 88 months later, the AI fails.
It’s the rule, not the exception, is that once deployed, your AI won’t work as expected. But think twice before putting all the blame on the Product Manager and the AI experts! Today, the process of deploying, training and testing AI is cumbersome, and it’s critical to get into it with as good of a system as possible in order to not waste precious time and money.
Only early deployment and learning in the relevant environment, in a continuous feedback loop does it enable us to truly build a (natural or artificial) intelligence capable to cope with reality vs our imaginary conception of it.
I learned the hard way… building and deploying AI for robots. I thought spending a ton of time devising a bullet-proof AI before seeing if it works in the field would work best. After a few failed attempts, my colleagues and I had to change strategy. Now, we focus on rapid prototyping before launching in the field. The sooner we can understand potential flaws, the better we’re able to address them.
So, deploy and keep learning, day after day. Like us, AI should never stop learning!